In today’s fast-evolving tech world, machine learning (ML) and deep learning (DL) are two essential forces driving artificial intelligence (AI) innovations. With the AI market projected to grow at a rate of 38% annually, reaching over $1.5 trillion by 2030, according to Precedence Research. That’s why understanding the difference between ML and DL has never been more important. While both involve algorithms that “learn” from data, deep learning’s specialized, layered approach allows it to tackle complex tasks that traditional machine learning models might struggle with.
This blog breaks down the unique strengths, data requirements, and computational demands of each, helping you grasp when and why to choose one over the other. Whether you’re a beginner exploring AI or a professional aiming to dive deeper, this guide will provide clear insights into how these powerful technologies are shaping industries worldwide.
In this video, ‘Machine Learning vs. Deep Learning,’ explore the core differences, strengths, and applications of these two transformative AI fields. This video breaks down their unique approaches, providing a clear guide for anyone interested in understanding how ML and DL shape the future of artificial intelligence.
What is the Difference Between Machine Learning and Deep Learning?
This table highlights the key distinctions between machine learning and deep learning, comparing their definitions, data requirements, feature engineering needs, computing power, accuracy, complexity, and ideal use cases.
Aspect | Machine Learning | Deep Learning |
Definition and Scope | Focuses on training algorithms to learn from data without explicit programming. | Subset of ML, uses artificial neural networks to mimic the human brain’s structure for complex pattern recognition. |
Data Requirements | Effective with smaller, structured datasets. | Requires large volumes of data, often unstructured, to perform well. |
Feature Engineering | Relies on manual feature engineering by data scientists to select and design the best features. | Automatically discovers features within data through layered neural networks, reducing the need for manual feature engineering. |
Computing Power | Requires less computing power; can be run on standard machines. | Requires substantial computing power, often needing GPUs or cloud-based solutions due to network complexity. |
Accuracy and Complexity | Works well for simpler tasks; sufficient for problems where deep relationships aren’t crucial. | Achieves high accuracy by capturing deep and complex relationships, ideal for advanced tasks like image and language processing. |
When to Use | Best for structured data and straightforward tasks, such as predictive analytics and classification. | Suited for tasks with unstructured data, like text, image, or audio processing, where deep pattern recognition is needed. |
What is Machine Learning?
Machine learning is a form of artificial learning that takes place by imitating human learning techniques. For any child to learn to recognize objects/persons, no procedure is explained to that child to introduce the properties of the objects/persons in question and then decide what they are. This child is only shown more than one instance of these objects/persons. Then the human brain automatically starts to identify these features over time (consciously) and learns to recognize objects/ persons. The machine learning model does the same.
In short, machine learning is the science of behaving and learning like humans on computers without directly programming computers by giving human observations to them in the form of information and data. The more different examples the data set contains, the better the machine learning will be; otherwise, the learning will not be good.
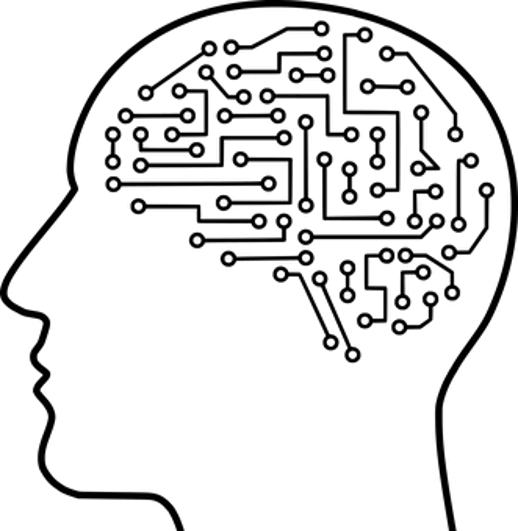
For example, a 30-year doctor has made so many diagnoses in his field throughout his professional life that he can quickly diagnose according to the test results. However, a 2-year doctor will not be able to diagnose comfortably because he is just beginning his professional life and perhaps will often need to consult the 30-year doctor.
The data set (experience) on the diseases in the brain of the 30-year doctor is so large that the brain can easily diagnose with a much higher accuracy rate based on past experiences, and it can easily understand which values in test results for diagnosis are important and which are not. But, since the data set (experience) of the 2-year doctor is small, it is natural that the accuracy rate of the disease diagnoses makes it much lower.
Two main approaches in machine learning are supervised and unsupervised learning. These are foundational methods that also appear in deep learning applications.
What is Supervised Learning in ML?
Supervised learning is a machine learning method where a model is trained on labeled data, meaning each data point includes input features along with its correct output label (or target value). This approach enables the model to learn the relationship between inputs and outputs, allowing it to make accurate predictions or classifications on new, unseen data.
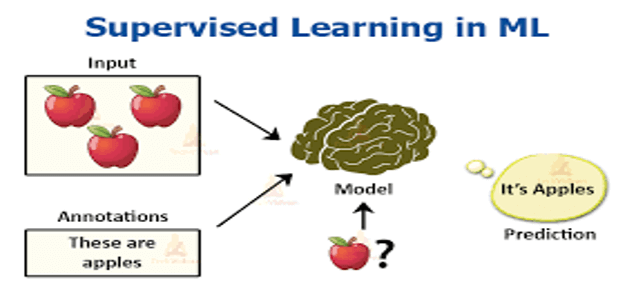
Supervised learning problems fall into two main categories:
- Regression: Predicts a continuous numeric value, such as house prices based on features like size and location.
- Classification: Predicts a category or label, like distinguishing between spam and non-spam emails.
In supervised learning, the model repeatedly adjusts its predictions based on errors to improve accuracy. For example, in cancer detection, a model can be trained on historical data that includes test values (inputs) and cancer diagnoses (outputs) to predict future cases with high accuracy.
Common supervised learning algorithms include:
- K-Nearest Neighbors
- Naive Bayes
- Linear Regression
- Logistic Regression
- Support Vector Machines (SVM)
- Decision Trees & Random Forests
- Neural Networks (some Neural networks architectures can be unsupervised)
What is Unsupervised Learning in ML?
Unsupervised learning is a machine learning method where a model is trained on unlabeled data—meaning it only has input data without corresponding output labels. The goal is for the model to identify patterns and organize the data into meaningful structures without prior guidance. This approach is commonly used in descriptive modeling and pattern detection.
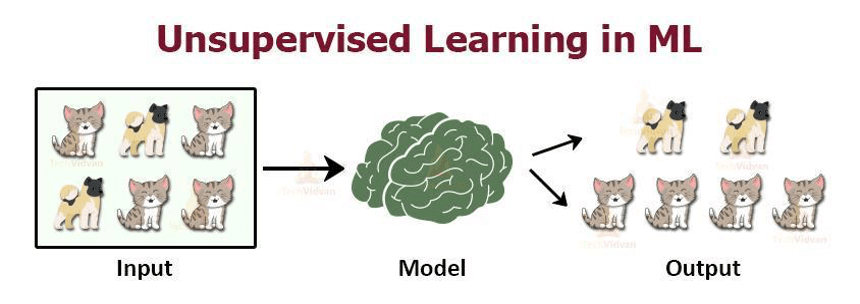
In unsupervised learning, two primary types of problems arise:
- Clustering: Grouping data points based on their similarities, such as segmenting customers into distinct groups.
- Association: Finding relationships between data points, such as market basket analysis in retail to see which products are often bought together.
Unsupervised learning is especially useful for exploring data when the patterns are unknown. For example, given a set of images without labels, the model might identify clusters based on shared features, grouping similar images together. While it cannot label these clusters (e.g., as “cats” or “dogs”), it can distinguish one group from another.
This method is widely applied in tasks like customer segmentation, anomaly detection, and recommendation systems.
The Quality Of The Data Set
The success of a machine learning algorithm is directly proportional to the quality of the data set as well as the selected algorithm. When we say quality, we mean the accuracy and completeness of the information in the data set. If the data given as input to the algorithm defines the output wholly and correctly, it will be a high-quality data set.
What is Deep Learning?
Deep learning is an advanced subset of machine learning that uses artificial neural networks to solve complex problems. Inspired by the structure of the human brain, deep learning models consist of multiple layers of interconnected “neurons” (small units of computation) that process data in stages, allowing the model to learn intricate patterns and representations.
A deep learning network typically has three main layers:
- Input Layer: Receives the raw data.
- Hidden Layers: These multiple layers perform calculations and extract features from the data. The deeper the network, the more hidden layers it has, enabling it to learn complex features.
- Output Layer: Produces the final prediction or classification.
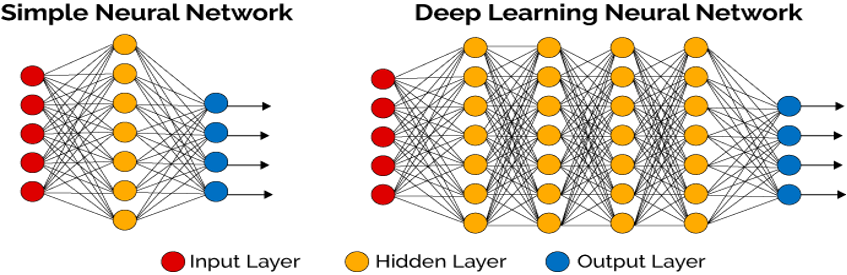
Training deep learning models requires substantial computing power and large amounts of labeled data, as the network needs to adjust its internal parameters across layers to minimize errors. This layered approach enables deep learning models to excel at tasks such as image recognition, natural language processing, and speech recognition, making it a powerful tool in artificial intelligence.
Artificial Neural Network
Let’s take a look at the deep learning working logic for the supervised learning method. Suppose we have a data set with price information according to car specifications (Brand, Model, Year of manufacture, Km, Horsepower). We want to make a car price estimation using this data set.
For estimating car prices, primarily the car specifications are given to the input layer. Since each car brand, car model, year of manufacture, Km, and Hp is a neuron, there are 5 neurons in the input layer. The input layer is responsible for sending these neurons (input data) to the first hidden layer.
In the hidden layer, various calculations are made with activation functions on the input data. The hidden layer(s) sends the values it finds resulting from these calculations to the output layer to make predictions. If the forecast is wrong in the output layer, the incoming values are sent back to the hidden layer to be calculated again. This cycle (called back-propagation) is repeated until it is realized with the smallest error.
The accuracy of the calculations performed in the hidden layer is directly proportional to the number of hidden layers and neurons that make up these layers. For this reason, this is one of the most important issues in deep learning.
What Are The Factors In Price Prediction For Deep Learning?
In the calculations made in the hidden layer, it is tried to determine how much the input data coming from the input layer affects the price estimation we will get from the output layer. A weight coefficient is assigned to each neuron according to its effect on the price estimation. This weighting coefficient is constantly updated until it reaches the most accurate estimate.
Hp has the largest weight coefficient in our example. So, it will have the biggest impact on the price prediction.
Training the Artificial Neural Network (ANN)
For the car price prediction, historical data of car prices are required. Therefore, we need a huge car price list due to many brand and model combinations.
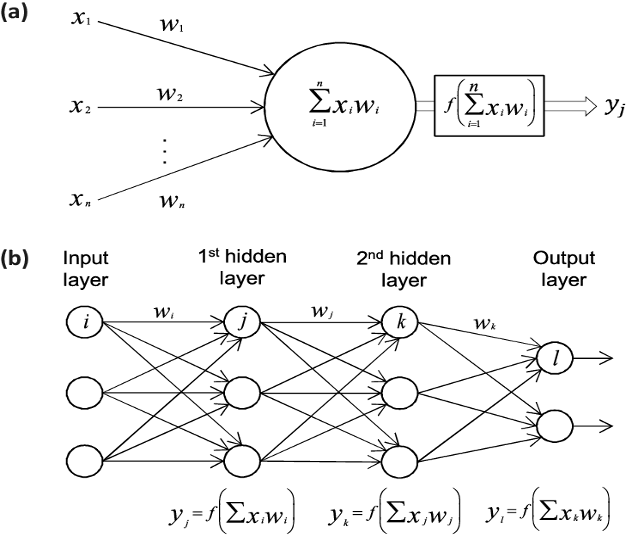
To train Artificial Neural Network (ANN), we need to give the inputs (car brand, car model, year of manufacture, Km and Hp) to ANN, and compare the outputs (estimated prices) we will get from ANN with the real prices in our dataset.
The function that calculates how estimated prices differ from actual prices is called the Cost (loss) Function. We want to do this during the training to reduce the value of the cost function to 0 (zero) as much as possible. In this way, we will be able to make the closest estimates to the real price.
How Can We Reduce The Cost (Loss) Function?
We can change the weights randomly until the cost function gets the closest value to zero, but this is not applicable as the training of the Artificial Neural Network requires huge computing power. Instead, we will use the Gradient Descent optimization algorithm, which does this automatically.
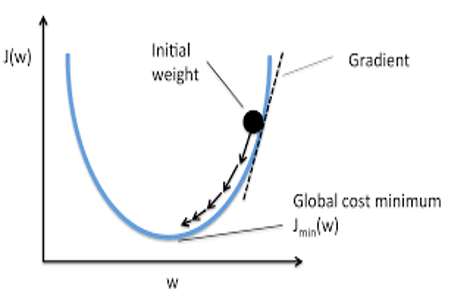
Gradient Descent works by changing the weights bit by bit throughout the backpropagation cycle. It determines the direction of the minimum point by calculating the derivative of the cost function in a certain set of weights. During training, the updated weight is referred to as the learning rate (step size).
When to Use Machine Learning vs Deep Learning?
The major advantage of deep learning is discovering hidden patterns in data and/or deeply understanding the complex relationships between variables and solving these complex problems. Deep learning algorithms detect and learn hidden patterns in the data by themselves and can create very efficient rules using these patterns.
For complex tasks that require dealing with a lot of unstructured data, such as natural language processing, speech recognition, or image classification, deep learning is always at the forefront. However, classical machine learning may be better suited for more straightforward tasks that require simpler feature engineering and do not require the processing of unstructured data.
Clarusway’s Machine Learning Course provides in-depth training in this fascinating field. Clarusway IT Bootcamp provides you with intense and realistic knowledge of using the system in real international situations using real world datasets. Through Machine Learning training, you will use performance metrics to evaluate and update machine learning models in a production environment.