In today’s technology-driven world, machine learning plays a critical role in shaping innovation and advancing what computers can achieve. It empowers systems to learn from data, understand patterns, and improve performance over time without explicit programming.
Machine learning emerged in the 1950s, pioneered by visionary thinkers like Arthur Samuel. It has since grown into a transformative field, revolutionizing industries such as finance, healthcare, and engineering. This blog explores its foundational principles, key approaches like supervised and unsupervised learning, and diverse applications.
Whether you are new to machine learning or looking to deepen your understanding, this guide will help you prepare for its real-world uses and uncover the vast opportunities it offers.
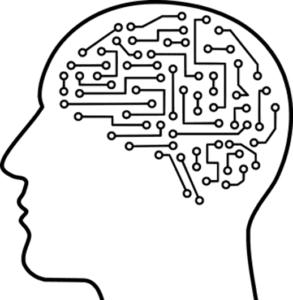
What is Machine Learning?
MMachine learning is a method that allows computers to learn patterns from data and make predictions or decisions. It involves training algorithms on datasets, enabling the system to adjust and improve over time. The process includes:
- Data Preprocessing: Cleaning and preparing data for analysis.
- Feature Selection: Identifying the most relevant data attributes.
- Model Training: Applying algorithms to datasets to find patterns.
- Model Testing: Evaluating accuracy using separate test data.
This iterative process improves predictions in applications like fraud detection, recommendation systems, and diagnostics.
Why is Machine Learning so Important?
Machine learning is important because it:
- Identifies trends in consumer behavior and business operations.
- Helps businesses uncover opportunities and mitigate risks.
- Analyzes complex and large datasets quickly and efficiently.
Is Machine Learning and Deep Learning Artificial Intelligence?
Yes, machine learning and deep learning are subfields of artificial intelligence (AI):
- Artificial Intelligence (AI): Broadly simulates human intelligence in machines to perform tasks.
- Machine Learning (ML): Develops algorithms that allow computers to learn from data and improve performance without explicit programming.
- Deep Learning (DL): A branch of ML that uses large datasets and complex algorithms to train models, focusing on tasks like image and speech recognition.
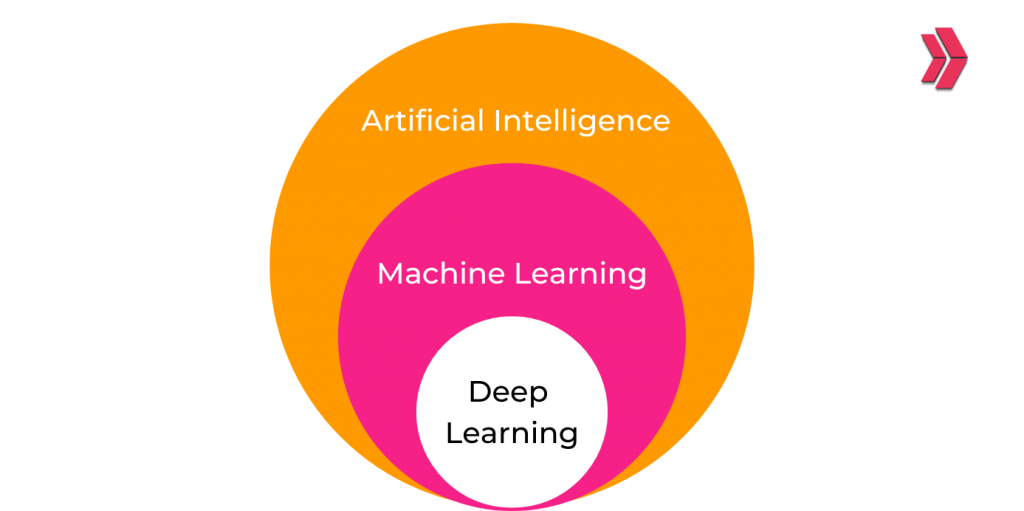
Is Machine Learning Data Science?
No, machine learning and data science are distinct fields:
- Data Science: Focuses on analyzing data to extract insights and meaning.
- Machine Learning: Develops methods to use data for predictions and improving performance.
Machine learning is one tool among many in the data scientist’s toolkit.
What are the Four Fundamentals of Machine Learning?
Machine learning has four primary strategies: supervised learning, unsupervised learning, semi-supervised learning, and reinforcement learning. These methods form the foundation for training algorithms to make decisions or predictions based on data.
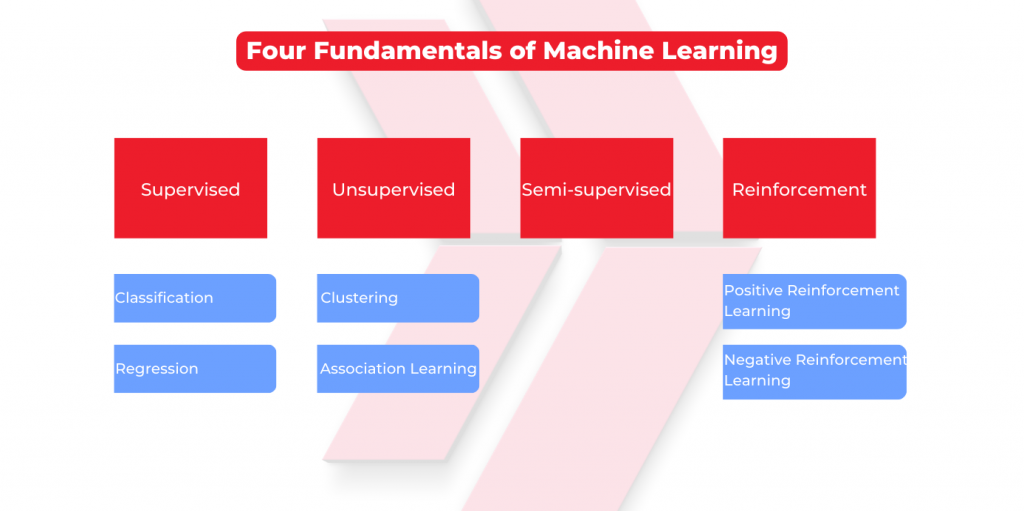
1. Supervised Machine Learning
SupSupervised machine learning trains models using labeled data, where each input is paired with a known output, also called a label or target. This method helps algorithms learn patterns and relationships to make accurate predictions or decisions.
How It Works
- Model Improvement: Through repeated adjustments, the algorithm becomes better at making predictions for new data.
- Training with Examples: The model learns from labeled data, identifying patterns between inputs and outputs.
- Error Correction: It compares predictions to the actual labels, identifies errors, and adjusts to improve accuracy.
In this video, Professor Eric Grimson from MIT introduces machine learning and shows examples of supervised learning.
Categories of Supervised Learning:
- Classification
Classification assigns data points to specific categories.
- Example: Sorting emails into “spam” or “not spam.”
- Use Case: Businesses use classification to analyze customer feedback or detect anomalies in data.
- Regression
Regression predicts continuous numerical values.
- Example: Predicting real estate prices based on property features.
- Use Case: Financial forecasting or predicting trends over time.
Popular Algorithm: Support Vector Machines (SVMs) are widely used for both classification and regression tasks.
Supervised learning is commonly applied in fraud detection, medical diagnostics, and recommendation systems. Its versatility makes it a cornerstone of machine learning for solving real-world problems.
2. Unsupervised Machine Learning
Unsupervised machine learning analyzes data to uncover patterns, relationships, or structures without the use of labeled outputs or specific guidance. Unlike supervised learning, which relies on labeled examples, unsupervised learning focuses on understanding the inherent structure within raw data.
How It Works
- Pattern Discovery: Algorithms scan datasets to identify meaningful patterns or groupings.
- Structure Analysis: It organizes data based on similarities or relationships without predefined categories.
- Applications: Common uses include customer segmentation, market analysis, and web usage mining.
Key Techniques in Unsupervised Learning
- Clustering
Clustering groups similar data points into clusters based on shared characteristics.
- Example: Grouping customers with similar purchasing habits for targeted marketing.
- Goal: Find natural groupings within data to better understand underlying patterns.
- Association Learning
Association learning identifies relationships or co-occurrence patterns within datasets.
- Example: Discovering that customers who buy bread also often buy butter (market basket analysis).
- Goal: Detect dependencies among items to optimize recommendations or inventory management.
Unsupervised learning is essential for tasks like customer behavior analysis, image recognition, and anomaly detection. It provides valuable insights when labeled data is unavailable, making it a powerful tool for exploratory data analysis.
3. Semi-supervised Learning
Semi-supervised learning combines aspects of supervised and unsupervised learning. It uses a dataset with both labeled and unlabeled examples, leveraging the strengths of each approach to enhance model performance.
How It Works
- Using Labeled Data: The algorithm learns from labeled examples to understand patterns and relationships.
- Incorporating Unlabeled Data: Unlabeled data is then used to refine and generalize the model’s understanding.
- Balancing Efficiency: This approach reduces the need for extensive labeling, making it cost-effective for large datasets.
Applications
- Facial Recognition: Identifying faces in webcam images, where only a portion of the data is labeled.
- Document Classification: Categorizing documents with minimal labeled examples.
Semi-supervised learning is particularly useful in scenarios where labeling data is expensive or time-consuming, offering a balance between accuracy and efficiency.
4. Reinforcement Learning
Reinforcement learning is a machine learning approach where an agent learns to take actions in an environment to maximize cumulative rewards. The learning process is based on trial and error, with the agent receiving feedback in the form of rewards or penalties for its actions.
How It Works
- Environment Interaction: The agent performs actions within an environment.
- Feedback Mechanism: Rewards are given for correct actions, while penalties are applied for incorrect ones.
- Learning Through Trial and Error: The agent refines its strategy based on past experiences to improve future performance.
The ultimate goal is to develop an optimal policy—a set of rules guiding the agent to achieve the best possible outcome.
Types of Reinforcement Learning
- Positive Reinforcement
Positive reinforcement strengthens desired behavior by adding a rewarding stimulus after a specific action.
- Example: A robot receives a reward for successfully navigating an obstacle.
- Negative Reinforcement
Negative reinforcement encourages desired behavior by removing an adverse condition after the action.
- Example: A system reduces penalties when an agent avoids making errors.
Reinforcement learning is widely applied in fields like robotics, game AI, autonomous vehicles, and recommendation systems. It excels in dynamic environments where the best actions are learned through ongoing interaction and feedback.
What are the Advantages of Machine Learning?
Listed below are some key advantages of Machine Learning:
1. Automates Processes
Machine learning enables computers to analyze data and generate accurate predictions without human intervention. Algorithms adapt over time to deliver reliable outcomes efficiently.
2. Versatile Applications
Machine learning is applied in diverse fields such as:
- Education: Enhancing e-learning platforms with personalized content.
- Medicine: Supporting diagnostics and drug discovery.
- Engineering: Optimizing processes and predictive maintenance.
It scales from small projects to large, complex systems, providing solutions tailored to different needs.
3. Handles Complex Data
Machine learning thrives in dynamic environments, managing large and varied datasets effectively. It can analyze diverse data sources simultaneously, making it ideal for multitasking.
4. Supports Continuous Improvement
As systems and professionals use machine learning, they refine their skills and outputs, driving efficiency and innovation. Companies like Google and Apple use this iterative process to stay at the forefront of technology.
5. Identifies Patterns and Trends
With increasing data, machine learning excels at uncovering patterns and trends, enabling better decision-making in fields like finance, marketing, and research.
6. Enhances Education and E-commerce
Machine learning supports:
- Education: Enabling online courses and personalized learning experiences.
- E-commerce: Providing targeted recommendations and real-time insights for consumers.
These advancements keep users informed and engaged in their respective domains.
Machine learning’s ability to automate, adapt, and provide insights ensures its value across industries, driving innovation and enhancing efficiency.
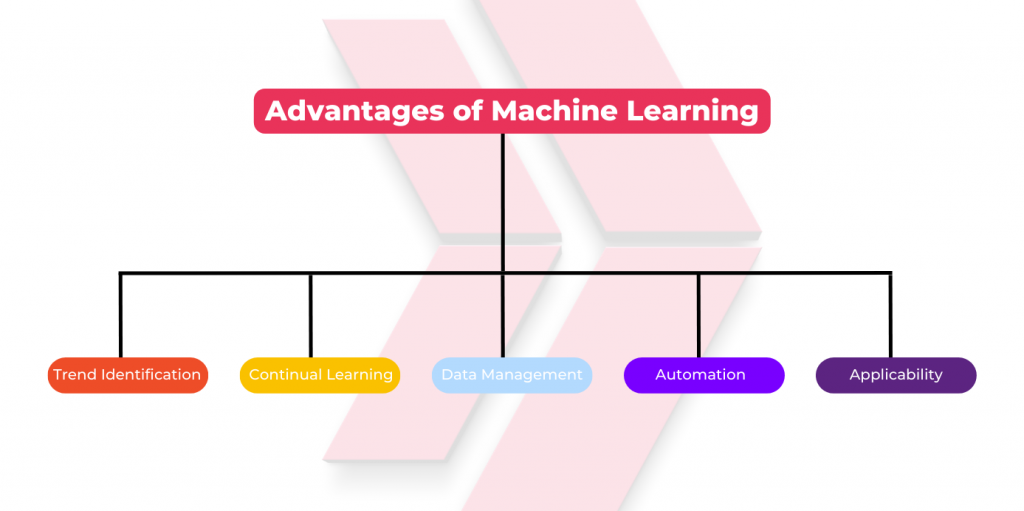
Who’s the User of Machine Learning?
Machine learning serves a diverse group of professionals and industries, enabling them to solve problems, make data-driven decisions, and optimize processes. Key roles and their applications include:
Machine Learning Engineer
Machine learning engineers design, build, and deploy models to handle large, complex datasets. They optimize data pipelines and ensure models operate efficiently in real-world environments.
Robotics Engineer
A Robotics Engineer employs machine learning to develop and improve computer vision systems that enable robots to process and interpret vast amounts of visual data. This enables robots to navigate, interact, and make decisions in real-world environments, enhancing their autonomy and capabilities.
Natural Language Processing (NLP) Scientist
A natural language processing scientist utilizes algorithms to determine the rules that make up the language in order to enable computers to speak and interpret natural language. Take a peek at ChatGTP to see this in action. The NLP Scientist uses computers to “understand, analyze, and manipulate human language.” Bridges the gap between human communication and machine comprehension using computer science and computational linguistics.
Software Developer
Software developers create mobile and desktop applications as well as basic operating systems. They utilize machine learning to analyze data and predict how customers will react to specific features of an application.
Software Engineer
A Software Engineer utilizes machine learning to analyze and predict user behavior, enabling the creation of more personalized and efficient applications. They integrate machine learning algorithms into software systems to enhance features like recommendation engines, fraud detection, and user interaction, leading to improved user experiences and outcomes.
Data Scientist
Data scientists use machine learning and predictive analytics to gather, analyze, and interpret large amounts of data in order to help companies make better decisions, optimize operations, and improve products. Machine learning is used more in data science jobs than in other fields.
Human-Centered Machine Learning Designer
A Human-Centered Machine Learning Designer is responsible for creating an information system that enables humans to interact with machines in an intuitive, productive, and meaningful way. To answer inquiries and solve issues, these specialists use human behavior and data-driven forecasts. Their responsibilities include developing AI-based technologies and designing apps and solutions with programming abilities.
Computational Linguists
Computational linguists work on developing ML systems capable of doing speech recognition, machine translation, and text mining. They create these systems from start to finish, collaborating with engineers to create software that is compatible with human language. They must be proficient in data analysis, natural language processing (NLP), Python, Java, Linux, and other programming languages.
Cybersecurity Analyst
Cybersecurity analysts are responsible for determining the best strategies to protect a company’s digital infrastructure and assets. This requires the use of several technologies, which may be greatly simplified by machine learning. This is due to the fact that a Cybersecurity Analyst is required to gather and analyze enormous volumes of data that indicate the vulnerabilities and dangers that a firm may face.
Artificial Intelligence (AI) Engineer
An Artificial Intelligence (AI) Engineer employs machine learning to develop intelligent systems that can perform tasks similar to human intelligence. They use machine learning algorithms to enable AI systems to learn from data, make predictions, recognize patterns, and adapt their behavior, leading to the creation of advanced AI applications and technologies.
Which Industries Use Machine Learning?
Machine learning drives innovation across various industries by enabling real-time data analysis, automating processes, and uncovering valuable insights. Below are some key sectors and examples of machine learning applications:
Social Media
The social community makes use of machine learning to apprehend acquainted faces in customers’ touch lists and allows automatic tagging. With ML, billions of customers can effectively interact on social media networks. Additionally, it is pivotal in using social media structures, from personalizing information feeds to turning in user-unique ads.
Speech Recognition
In speech recognition, machine learning enables devices and systems to accurately transcribe spoken language into text, facilitating voice commands and interactions.
Customer Service
In customer service, machine learning enhances support interactions by automating responses, analyzing sentiment, and providing personalized recommendations to improve user experiences.
Computer Vision
This AI generation permits computer systems to derive significant statistics from virtual pictures, motion pictures, and different virtual inputs.
Recommendation Engines
The recommendation engines at the back of Netflix and YouTube suggestions, what statistics seem for your Facebook feed, and product guidelines are fueled by machine learning.
Automated Inventory Buying and Selling
The modern-day era of AI-pushed high-frequency buying and selling structures makes hundreds or maybe hundreds of thousands of trades in step with the day without human intervention.
Fraud Detection
Machine learning is being used inside the monetary and banking zone to autonomously examine large numbers of transactions to discover fraudulent pastimes. Anomaly detection can discover transactions that appear odd and deserve a similar investigation. Machines can examine styles, like how a person generally spends or wherein they generally shop, to discover fraudulent credit score card transactions.
Medical Imaging and Diagnostics/Healthcare Industry
Machine learning is being increasingly followed inside the healthcare enterprise, from credit scores to wearable gadgets and sensors, including wearable health trackers, clever fitness watches, etc. Additionally, the technology is supporting scientific practitioners for;
- Affected person diagnoses
- Treatment
- Drug discovery
- Customized treatment
- Boost up the discovery of remedies and cures
- Enhance affected person outcomes
- Automate ordinary strategies to save you from human error
Image Evaluation and Item Detection
Machine learning can examine photos for specific information, like studying to perceive human beings and inform them apart — even though facial reputation algorithms are controversial.
Data Security
By searching beyond experiences, machine learning models can predict expected destiny in high-danger sports so that danger may be proactively mitigated.
Finance
Banks, buying and selling brokerages, and fintech corporations use machine learning algorithms to automate buying and selling and to offer economic advisory offerings to traders to determine while to trade.
Retail
Retailers use machine learning strategies for;
- Applicable product suggestions.
- Advertising campaigns.
- Consumer insights.
- Consumer products planning.
- Rate optimization.
- Digital assistants or conversational chatbots.
Primarily based on buyers’ purchase histories and historical, geographic, and demographic facts.
Travel Enterprise
The journey enterprise makes use of system studying to research personal feedback;
- Classify advantageous or bad scores.
- Marketing campaign monitoring.
- Emblem monitoring.
- Compliance monitoring.
Government
Government companies, including public protection and utilities, use machine learning for;
- Insights.
- Identifies methods to boost efficiency.
- Store money.
- Come across a fraud.
- Decrease identification theft.
Oil and Gasoline
Oil and fuel line zones use machine learning for;
- Locating new sources of power.
- Analyzing the ground’s mineral composition.
- Predict sensor failure at a refinery.
Transportation
Public transportation and different transportation agencies use machine learning for;
- Making routes extra efficient.
- Predict capacity troubles to boom profitability.
How to Study Machine Learning?
To effectively study machine learning and prepare for a career in this field, consider the following steps:
- Build a Strong Foundation:
- Start by understanding the basics of programming, mathematics (linear algebra, calculus, and statistics), and data manipulation.
- Familiarize yourself with relevant programming languages like Python, which is widely used in the machine learning community.
- Learn the Fundamentals of Machine Learning:
- Begin with the fundamentals of machine learning concepts, including supervised and unsupervised learning, overfitting, underfitting, bias-variance trade-off, and cross-validation.
- Online Courses and Resources:
- Enroll in reputable online machine learning courses or programs, such as Clarusway’s Machine Learning Course, to get structured learning and hands-on experience.
- Utilize online resources like tutorials, blogs, and video lectures to deepen your understanding.
- Practice with Real-world Datasets:
- Work on real-world datasets to gain practical experience. Platforms like Kaggle offer datasets and competitions that allow you to apply machine-learning techniques.
- Explore Algorithms and Models:
- Learn about various machine learning algorithms and models, such as linear regression, decision trees, support vector machines, and neural networks.
- Understand when and how to use different algorithms for different types of problems.
- Implement Projects:
- Create your own machine-learning projects to apply what you’ve learned. Projects demonstrate your skills to potential employers and help solidify your understanding.
- Experiment and Iterate:
- Experiment with different algorithms, hyperparameters, and techniques to understand their impact on model performance.
- Iteratively refine your models based on insights gained from experimentation.
- Learn About Deep Learning :
- Delve into deep learning, a subfield of machine learning focused on neural networks and their applications.
- Stay Updated:
- Machine learning is a rapidly evolving field. Stay up-to-date with the latest advancements, research papers, and industry trends.
- Networking and Collaboration:
- Join machine learning communities, conferences, workshops, and meetups to connect with professionals in the field.
- Collaborate on open-source projects to enhance your skills and visibility.
- Build a Portfolio:
- Showcase your projects, code, and insights on platforms like GitHub. A strong portfolio demonstrates your expertise to potential employers.
- Seek Internships or Entry-level Positions:
- Apply for internships or entry-level positions in companies that use machine learning.
- Gain practical experience and learn from experienced professionals in a real-world setting.
- Continuous Learning:
- Machine learning is constantly evolving. Keep learning and adapting to new techniques and technologies.
Clarusway’s Machine Learning Course will put you on the path to success in this fascinating field. Clarusway IT Bootcamp offers you in-intensity and realistic know-how on using the system in actual international cases by using real-world datasets. Through Machine Learning training, you will use performance metrics to evaluate and update machine learning models in a production environment. Additionally, Clarusway provides various payment options to make the program work for you. Are you ready to give your career a boost?
FAQs
1. How is machine learning different from deep learning?
Machine learning is a method where algorithms learn patterns from data to make predictions or decisions. Deep learning, a subset of machine learning, uses neural networks to analyze large datasets and solve complex problems like image and speech recognition.
For a detailed comparison, check out our article:”Machine Learning vs. Deep Learning Explained“
2. What role does machine learning play in data science?
Machine learning is a key tool in data science. While data science focuses on analyzing and extracting insights from data, machine learning provides methods to create predictive models that enhance decision-making.
Learn more in our guide:”What is Data Science? A Beginner’s Guide To Data Science“
3. What are the most common questions about deep learning?
Deep learning often raises questions about its use cases, data requirements, and differences from other AI techniques. It is widely applied in fields like image recognition, language translation, and robotics.
Discover answers to these top questions: “Unlock the Power of Deep Learning: Top 3 Questions Answered“